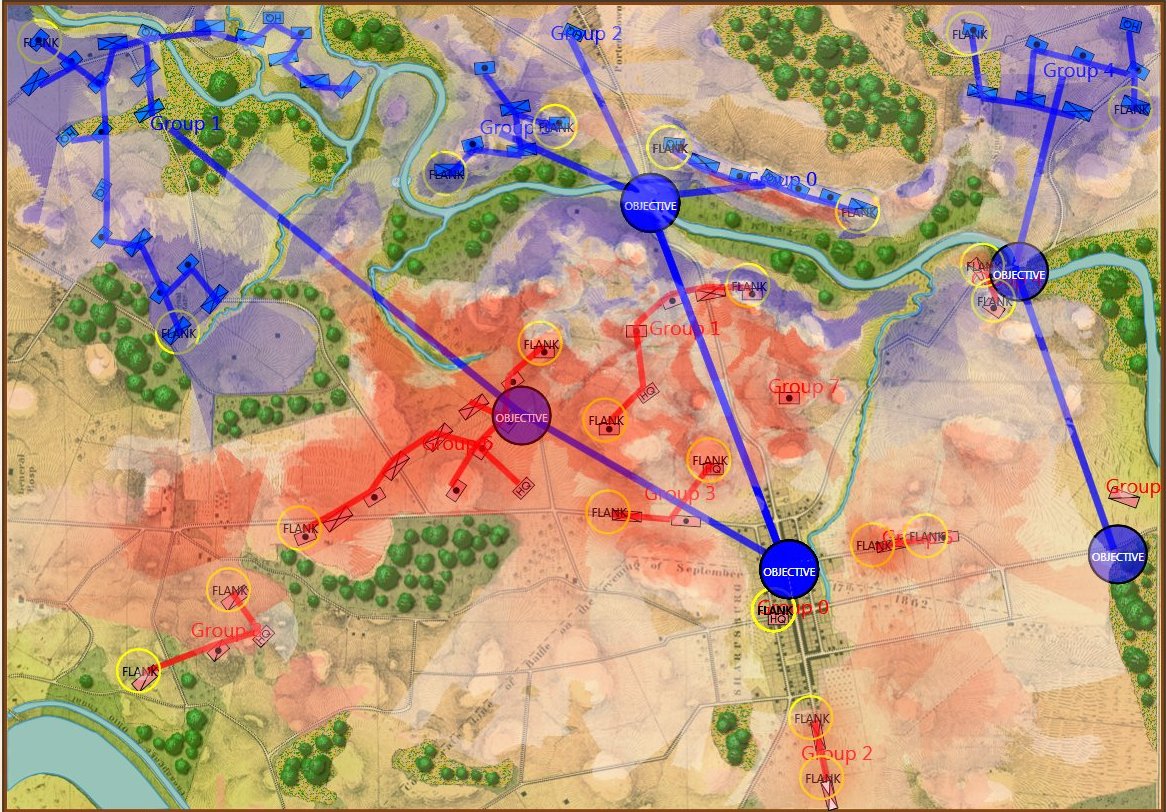
MATE AI selected Objectives for Blue, 3D Line of Sight (3DLOS) and Range of Influence (ROI) displayed for the Antietam: Dawn General Staff scenario. Screen shot from General Staff Sand Box. Click to enlarge.
I have been thinking about creating an artificial intelligence (AI) that could make good tactical decisions for the battle of Antietam (September 17, 1862, Sharpsburg, Maryland) for over fifty years. At the time there was little thought of computers playing wargames.1)However, it is important to note that Arthur Samuel had begun research in 1959 into a computer program that could play checkers. See. “Samuel, Arthur L. (1959). “Some Studies in Machine Learning Using the Game of Checkers”. IBM Journal of Research and Development.” What I was envisioning was a board wargame with some sort of look-up tables and coffee grinder slide rules that properly configured (not sure how, actually) would display what we now call a Course of Action (COA), or a set of tactical orders. I didn’t get too far on that project but I did create an Antietam board wargame when I was 13 though it was hardly capable of solitaire play.
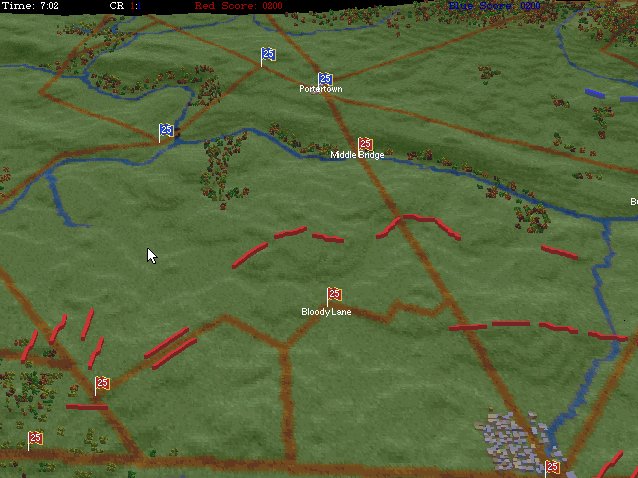
The Antietam scenario from The War College (1992). This featured 128 pre-rendered 3D views generated from USGS Digital Elevation Model Maps.
In 1992 I created my first wargame with an Antietam scenario: The War College (above). It used a scripted AI that isn’t worth talking about. However, in 2003 when I began my doctoral research into tactical AI I had the firm goal in my mind of creating software that could ‘understand‘ the battle of Antietam.
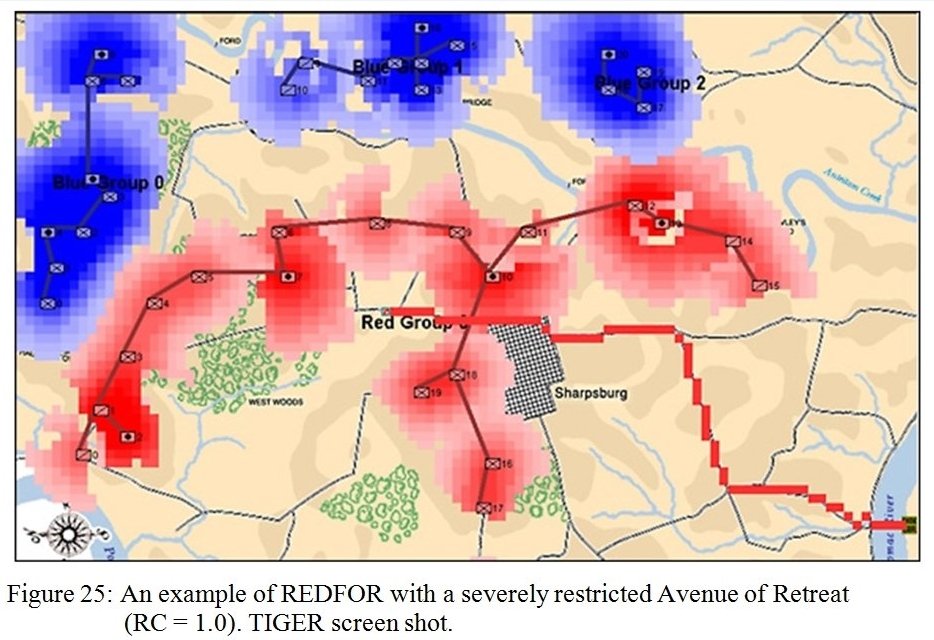
TIGER Analysis of the battle of Antietam showing Range of Influence of both armies, battle lines and RED’s avenue of retreat. TIGER screen shot. Appears in doctoral thesis, “TIGER: A Machine Learning Tactical Inference Generator,” University of Iowa 2009
The TIGER program met that goal (the definition of ‘understand’ being: performing a tactical analysis that is statistically indistinguishable from a tactical analysis performed by 25 subject matter experts; e.g.. active duty command officers, professors of tactics at military institutes, etc.).
In the above screen shot we get a snapshot of how TIGER sees the battlefield. The darker the color the greater the firepower that one side or the other can train on that area. Also shown in the above screen shot is that RED has a very restricted Avenue of Retreat; the entire Confederate army would have to get across the Potomac using only one ford (that’s the red line tracing the road net to the Potomac). Note how overlapping ROIs cancel each other out. In my research I discovered that ROIs are very important for determining how battles are described. For example, some terms to describe tactical positions include:
- Restricted Avenue of Attack
- Restricted Avenue of Retreat
- Anchored Flanks
- Unanchored Flanks
- Interior Lines
- No Interior Lines
Between the time that I received my doctorate in computer science for this research and the time I became a Principal Investigator for DARPA on this project the name changed from TIGER to MATE (Machine Analysis of Tactical Environments) because DARPA already had a project named TIGER. MATE expanded on the TIGER AI research and added the concept of Predicate Statements. Each statement is a fact ascertained by the AI about the tactical situation on that battlefield. The most important statements appear in bold.
The key facts about the tactical situation at Antietam that MATE recognized were:
- REDFOR’s flanks are anchored. There’s no point in attempting to turn the Confederate flanks because it can’t be done.
- REDFOR has interior lines. Interior lines are in important tactical advantage. It allows Red to quickly shift troops from one side of the battlefield to the other while the attacker, Blue, has a much greater distance to travel.
- REDFOR’s avenue of retreat is severely restricted. If Blue can capture the area that Red must traverse in a retreat, the entire Red army could be captured if defeated. Lee certainly was aware of this during the battle.
- BLUEFOR’s avenue of attack is not restricted. Even though the Blue forces had two bridges (Middle Bridge and Burnside’s Bridge) before them, MATE determined that Blue had the option of a wide maneuver to the north and then west to attack Red (see below screen shot):
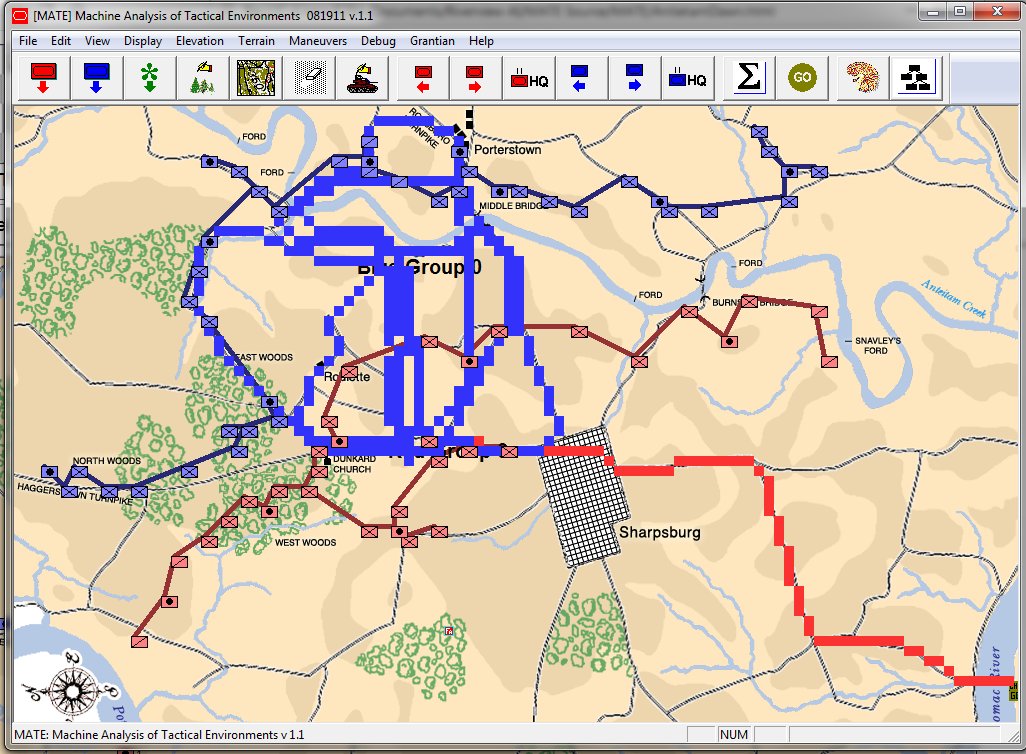
MATE analysis shows that Blue units are not restricted to just the two bridge crossings to attack Red. MATE screen shot.
- BLUEFOR has the superior force. The Union army was certainly larger in men and materiel at Antietam.
- BLUEFOR is attacking across level ground. Blue is not looking at storming a ridge like at the battle of Fredericksburg.
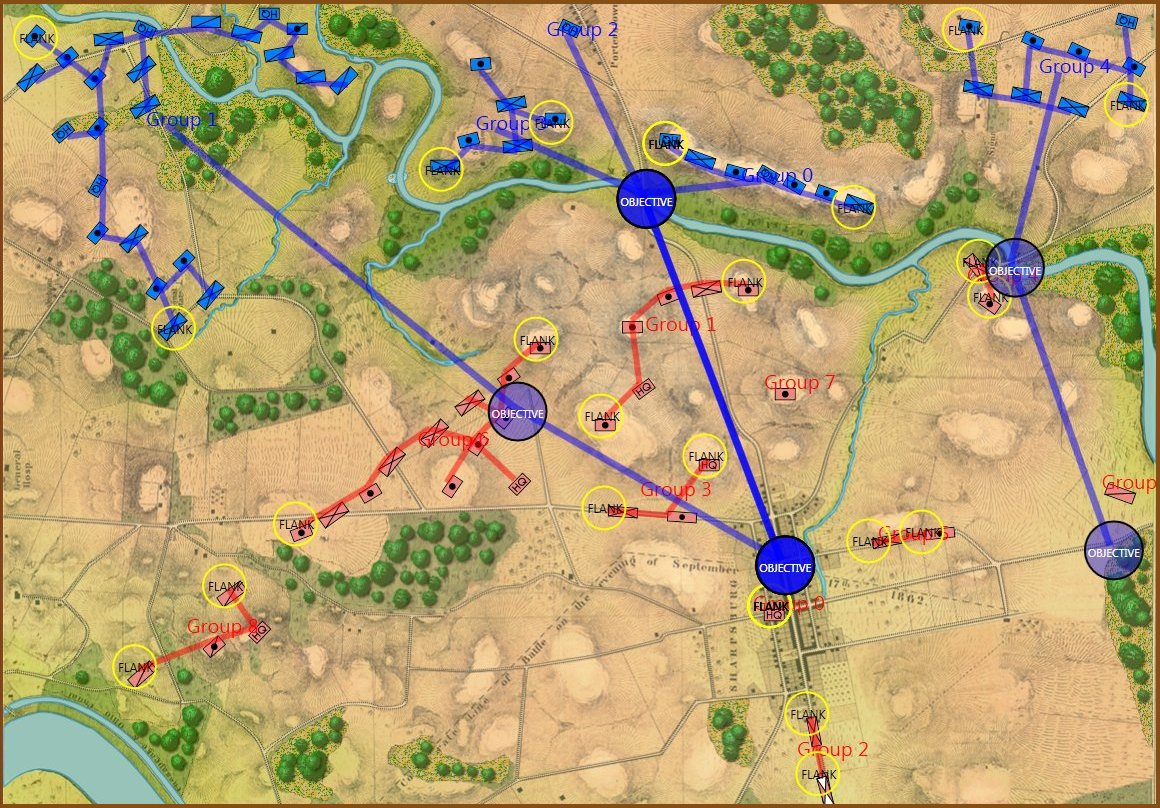
MATE AI selects these objectives for Blue’s attack. General Staff Sand Box screen shot. Click to enlarge.
We now come to General Staff which uses the MATE AI. General Staff clearly has a much higher resolution than the original TIGER program (1155 x 805 terrain / elevation data points versus 102 x 66, or approximately 138 times the resolution / detail). In the above screen shot the AI has selected five Objectives for Blue. I’ve added the concept of a ‘battle group’ – units that share a contiguous battle line – which in this case works out as one or two corps. Each battle group has been assigned an objective. How each battle group achieves its objective is determined by research that I did earlier on offensive tactical maneuvers 2)See, “Implementing the Five Canonical Offensive Maneuvers in a CGF Environment.” link to paper.
As always, I appreciate comments and questions. Please feel free to email me directly with either.
References
↑1 | However, it is important to note that Arthur Samuel had begun research in 1959 into a computer program that could play checkers. See. “Samuel, Arthur L. (1959). “Some Studies in Machine Learning Using the Game of Checkers”. IBM Journal of Research and Development.” |
---|---|
↑2 | See, “Implementing the Five Canonical Offensive Maneuvers in a CGF Environment.” link to paper. |